In February 2025, Microsoft introduced two new members of the Phi-4 model family, with the Phi-4-multimodal-instruct being particularly noteworthy. Despite having just 5.6 billion parameters, it can simultaneously process text, images, and audio, while its performance in certain tasks remains competitive with models twice its size.
The Phi-4-multimodal-instruct was developed using an innovative "Mixture of LoRAs" technique, which allows the base model to be extended with audio and visual capabilities without requiring a full retraining of the model, thus minimising interference between different modalities. The model boasts a context length of 128,000 tokens. On the Hugging Face OpenASR leaderboard, it achieves a word error rate of 6.14%, securing the top spot and surpassing the specialised WhisperV3 speech recognition system. According to Weizhu Chen, Microsoft’s Vice President of Generative AI, these models are designed to equip developers with advanced AI capabilities. The model supports speech recognition in eight languages, including English, Chinese, German, French, Italian, Japanese, Spanish, and Portuguese, while handling 23 languages in text format.
The Phi-4-multimodal-instruct and the 3.8 billion-parameter Phi-4-mini are already available on the Hugging Face platform under an MIT licence, which also permits commercial use. The Phi-4-mini delivers particularly outstanding performance in mathematical and coding tasks, achieving an 88.6% score on the GSM-8K maths test and 64% on the MATH benchmark, significantly outperforming competitors of similar size. Capacity, an AI-based enterprise software development company, has already integrated the Phi model family into its systems. According to the company’s report, using the Phi models resulted in a 4.2-fold cost reduction compared to competing solutions, while delivering equal or better quality results in preprocessing tasks. Microsoft’s statement highlights that these models can run not only in data centres but also on standard hardware or directly on devices, significantly reducing latency and data privacy risks.
Sources:
1.
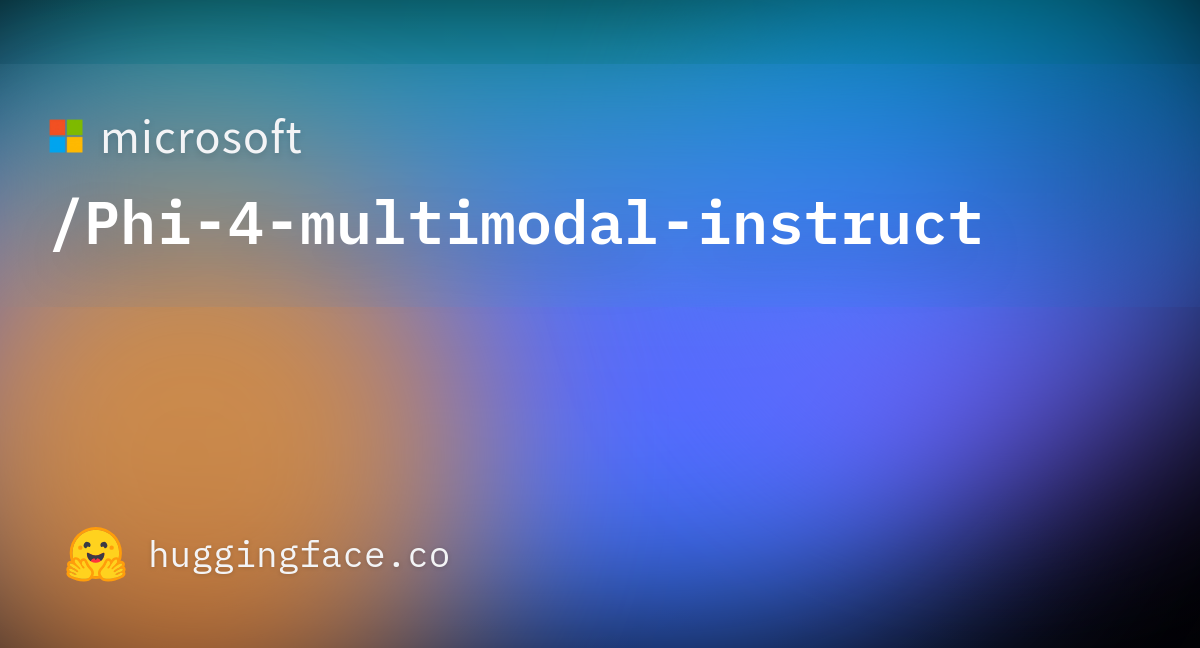
2.
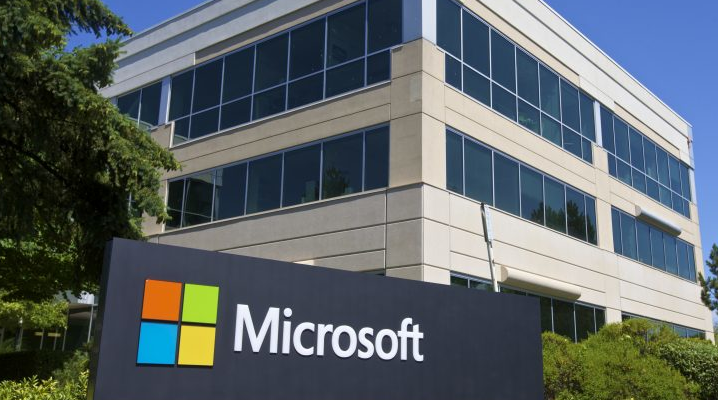
3.
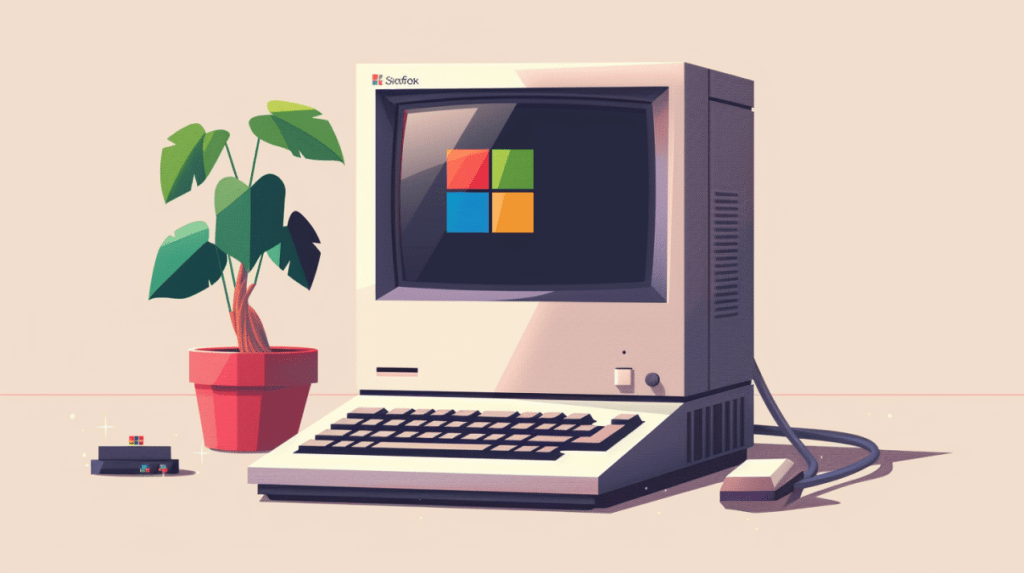