The concept of generative artificial intelligence (GenAI) has long been employed in academic and technological discourse; however, it only began to garner widespread recognition in the mid-to-late 2010s, a period marked by pivotal breakthroughs in AI development that fundamentally transformed the landscape of its potential applications. Early in the decade, significant advances in machine learning (ML) and artificial neural networks (ANNs) (LeCun, Bengio, & Hinton 2015) opened up new horizons in areas such as computer vision (Krizhevsky, Sutskever, & Hinton 2013) and natural language processing (NLP) (Vaswani et al. 2017). According to Bill Gates, founder of Microsoft, these tools represent the most important technological advance since the advent of the graphical user interface in the 1980s (Gates 2023).
One of the earliest milestones in this wave of breakthroughs was the introduction of Generative Adversarial Networks (GANs) by Ian Goodfellow in 2014 (Goodfellow et al. 2014), which enabled models to generate new data—such as photorealistic images—based on existing patterns. In 2017, a team of researchers at Google led by Ashish Vaswani unveiled the Transformer architecture (2017), which fundamentally reshaped the fields of natural language processing and generative technologies. The Transformer, built on self-attention mechanisms, overcame the limitations of earlier recurrent neural networks (RNNs, Mikolov et al. 2013) and rendered the processing and generation of information much more efficient and scalable. This innovation laid the foundation for the subsequent development of generative language models, most notably the GPT series.
In 2018, OpenAI introduced the Generative Pre-trained Transformer (GPT), a language model capable of identifying complex linguistic structures and patterns, as well as generating coherent and contextually appropriate texts, through training on an extensive and diverse corpus of textual data (Radford et al. 2018). This landmark development was followed in 2019 by GPT-2, a significantly more powerful model with billions of parameters. GPT-2 demonstrated the ability to produce sophisticated texts that were, in many cases, virtually indistinguishable from human-written content (Radford et al. 2019).
From the 2020s onwards, generative AI technologies became widely accessible, ushering in a new era in the practical applications of artificial intelligence. In August 2022, Stable Diffusion was released, enabling the generation of realistic and imaginative images from textual descriptions (Rombach et al. 2022). This was soon followed, in November 2022, by the release of ChatGPT, an interactive language model capable of real-time communication by generating text-based responses to user queries and prompts (OpenAI 2022). Owing to its advanced natural language processing capabilities, the chatbot can respond to complex questions, produce creative texts, and even generate code—thus revolutionising the deployment of generative AI. (The key milestones in the development of generative AI are summarised in the figure below.)
The release of OpenAI’s GPT-4 model (OpenAI 2023a), along with newly introduced functionalities—such as browser plug-ins (OpenAI 2023b and third-party extensions (custom GPTs)—further expanded the application potential of generative AI. ChatGPT rapidly emerged as one of the fastest-growing consumer applications, reaching one million users within just five days of its launch in November 2022 (Brockman 2022). As of the latest figures from December 2024, the platform boasts 300 million weekly active users (OpenAI Newsroom 2024). The developmental trajectory shaped by generative adversarial networks, Transformer architectures, and GPT models has produced generative systems that are now applicable across virtually all domains—from the creative industries to scientific research.
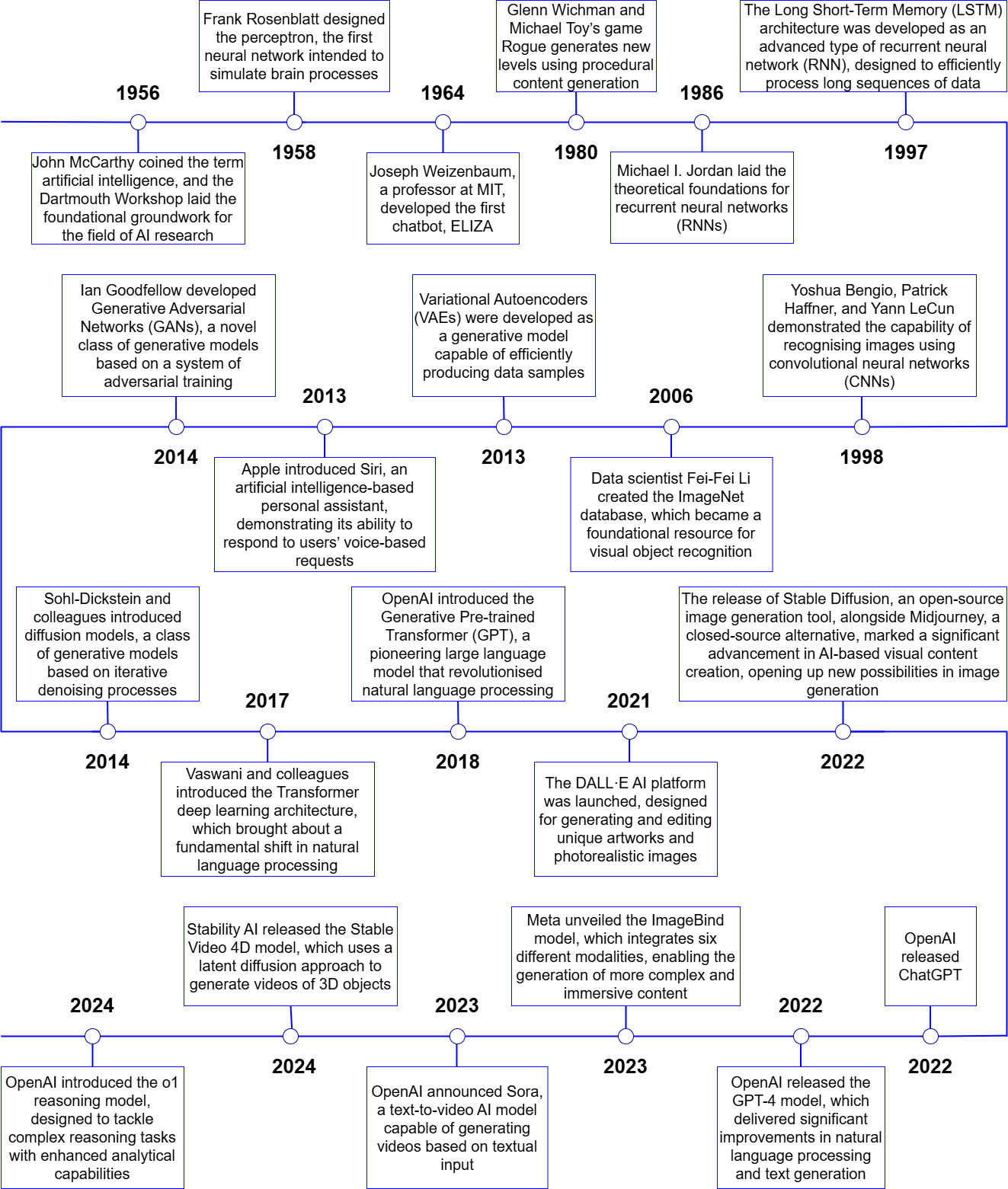
References:
1. Brockman, Greg. 2022. ‘ChatGPT Just Crossed 1 Million Users; It’s Been 5 Days since Launch.’ X. https://x.com/gdb/status/1599683104142430208 – ^ Back
2. Gates, Bill. 2023. ‘The Age of AI Has Begun’. GatesNotes. 21. – https://www.gatesnotes.com/The-Age-of-AI-Has-Begun – ^ Back
3. Goodfellow, Ian J., Jean Pouget-Abadie, Mehdi Mirza, Bing Xu, David Warde-Farley, Sherjil Ozair, Aaron Courville, and Yoshua Bengio. 2014. ‘Generative Adversarial Networks’. arXiv. doi:10.48550/ARXIV.1406.2661 – ^ Back
4. Krizhevsky, Alex, Ilya Sutskever, and Geoffrey E. Hinton. 2013. ‘ImageNet Classification with Deep Convolutional Neural Networks’. Advances in Neural Information Processing Systems 25: 26th Annual Conference on Neural Information Processing Systems 2012; December 3 - 6, 2012, Lake Tahoe, Nevada, USA, 1–9. – ^ Back
5. LeCun, Yann, Yoshua Bengio, and Geoffrey Hinton. 2015. ‘Deep Learning’. Nature 521 (7553): 436–44. doi:10.1038/nature14539 ^ Back
6. Mikolov, Tomas, Ilya Sutskever, Kai Chen, Greg Corrado, and Jeffrey Dean. 2013. ‘Distributed Representations of Words and Phrases and Their Compositionality’. arXiv. doi:10.48550/ARXIV.1310.4546 – ^ Back
7. OpenAI. 2022. ‘Introducing ChatGPT’. https://openai.com/index/chatgpt/ – ^ Back
8. OpenAI. 2023a. ‘GPT-4 Technical Report’. Download PDF – ^ Back
9. OpenAI. 2023b. ‘GPT-4 Technical Report’. Download PDF – ^ Back
10. OpenAI Newsroom. 2024. ‘Fresh Numbers Shared by @sama Earlier Today’. X. 04. https://x.com/OpenAINewsroom/status/1864373399218475440 – ^ Back
11. Radford, Alec, Karthik Narasimhan, Tim Salimans, and Ilya Sutskever. 2018. ‘Improving Language Understanding by Generative Pre-Training’. Download PDF – ^ Back
12. Radford, Alec, Jeffrey Wu, Rewon Child, David Luan, Dario Amodei, and Ilya Sutskever. 2019. ‘Language Models Are Unsupervised Multitask Learners’. Download PDF – ^ Back
13. Rombach, Robin, Andreas Blattmann, Dominik Lorenz, Patrick Esser, and Björn Ommer. 2022. ‘High-Resolution Image Synthesis with Latent Diffusion Models’. arXiv. doi:10.48550/ARXIV.2112.10752 – ^ Back
14. Vaswani, Ashish, Noam Shazeer, Niki Parmar, Jakob Uszkoreit, Llion Jones, Aidan N. Gomez, Lukasz Kaiser, and Illia Polosukhin. 2017. ‘Attention Is All You Need’. arXiv. doi:10.48550/ARXIV.1706.03762 – ^ Back