In March 2025, Sakana AI’s "AI Scientist-v2" system achieved a significant milestone by producing the first fully AI-generated scientific paper to successfully pass peer review at a workshop of the ICLR conference. The research team submitted three AI-generated studies, one of which—titled "Compositional Regularization: Unexpected Obstacles in Enhancing Neural Network Generalization"—received an average score of 6.33, surpassing the acceptance threshold, though it was ultimately withdrawn before publication.
The AI Scientist-v2 produced the study entirely autonomously, without human intervention: it formulated a scientific hypothesis, designed experiments, wrote and refined code, executed the experiments, analysed and visualised the data, and drafted the complete manuscript from title to references. This breakthrough demonstrates that artificial intelligence can independently generate research that meets the rigorous standards of peer review, according to the Sakana AI team. However, the system made some "embarrassing" citation errors, such as incorrectly attributing a method to a 2016 study instead of the original 1997 work. Additionally, the acceptance rate for workshop papers (60-70%) is higher than that for main conference submissions (20-30%), and Sakana admitted that none of the generated studies met their own internal quality threshold for publication at the leading ICLR conference.
The experiment was conducted in collaboration with researchers from the University of British Columbia and the University of Oxford, with the full support of the ICLR leadership and approval from the University of British Columbia’s Institutional Review Board (IRB). It raises crucial questions about the transparency, ethics, and future of AI-generated scientific publications. Sakana AI emphasised its commitment to maintaining an ongoing dialogue with the scientific community regarding the development of this technology, aiming to prevent a potential scenario where the sole purpose of AI-generated research becomes passing the peer-review process, which could significantly undermine the credibility of scientific peer evaluation.
Sources:
1.
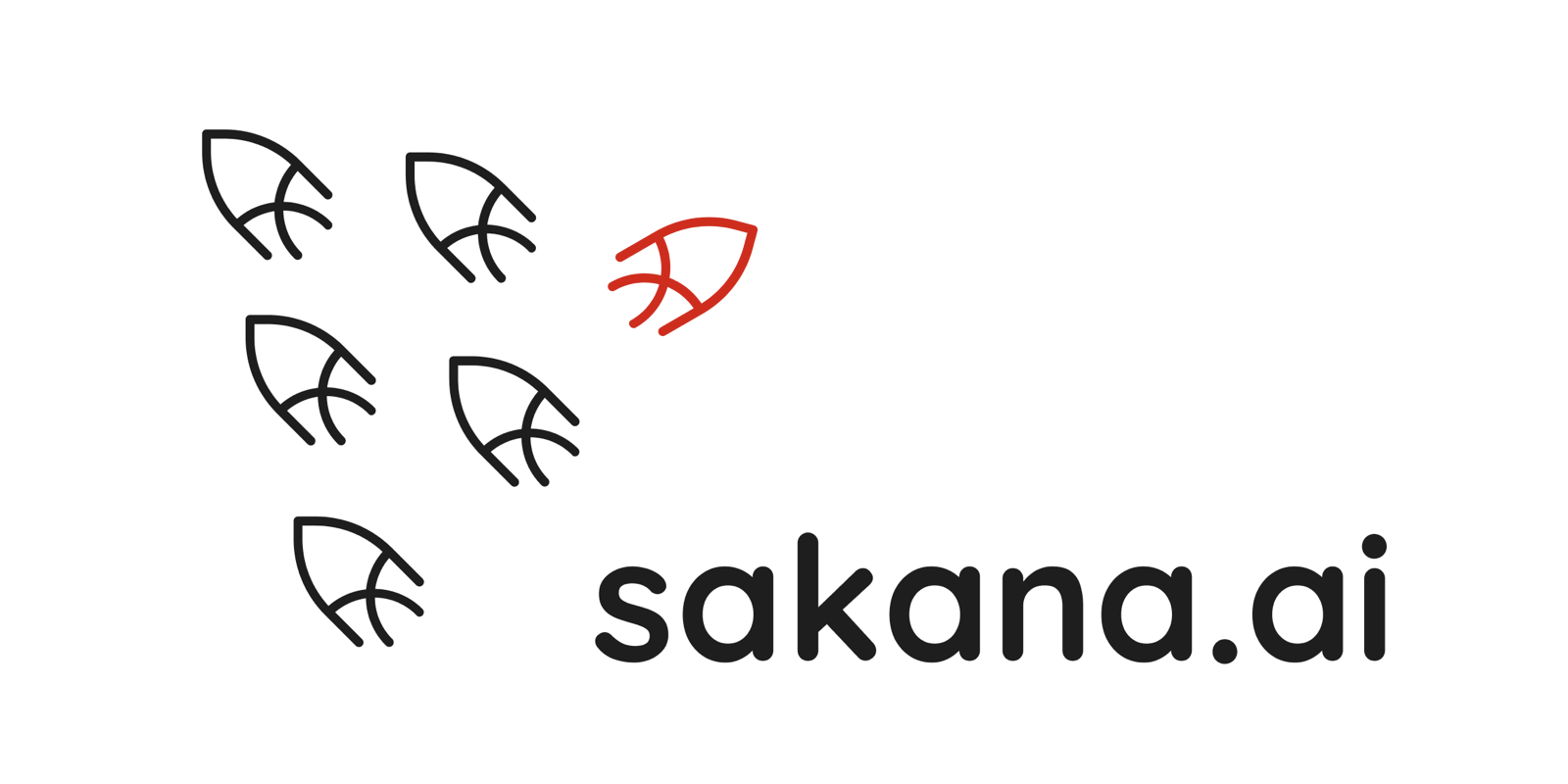
2.
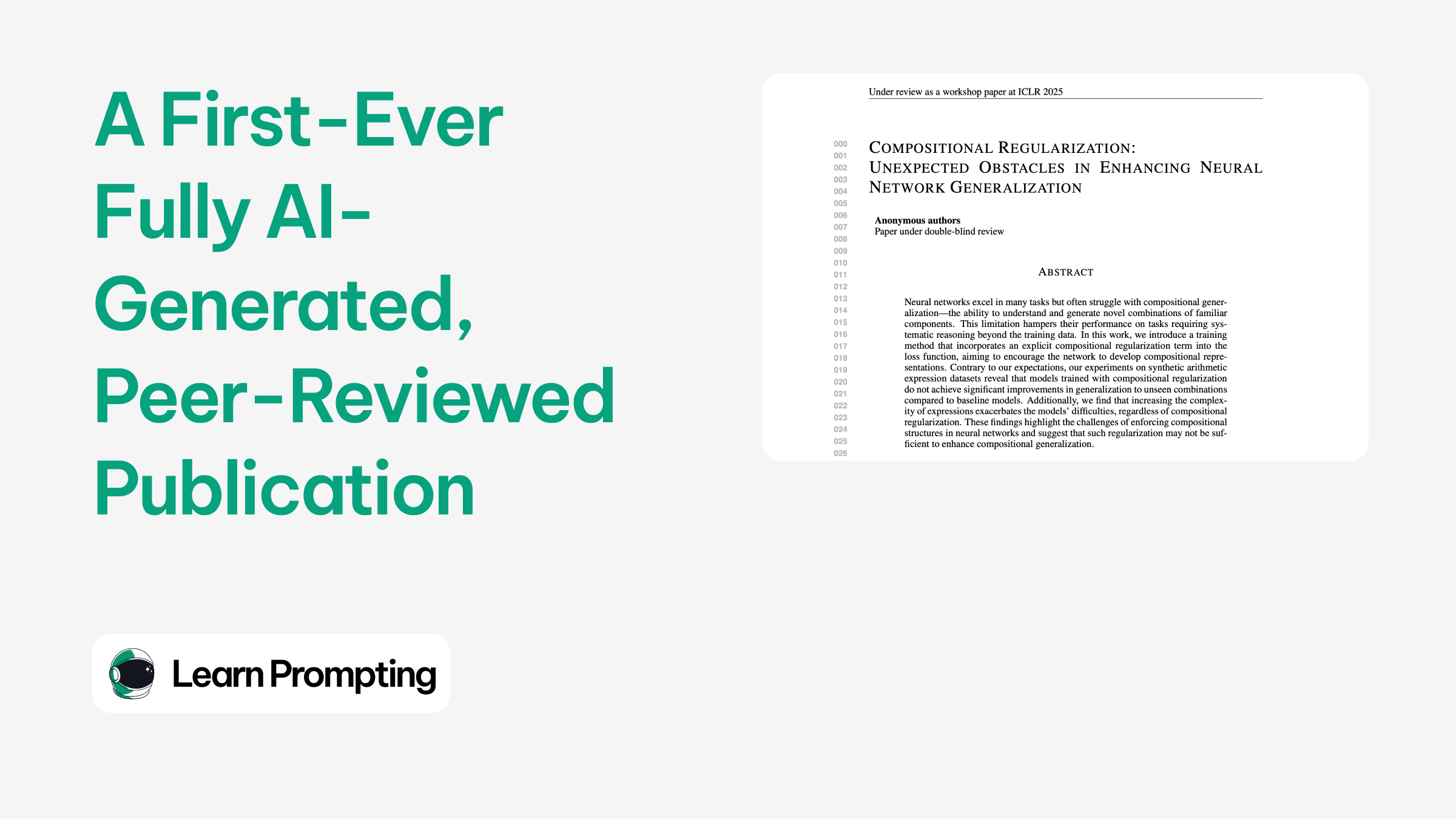
3.
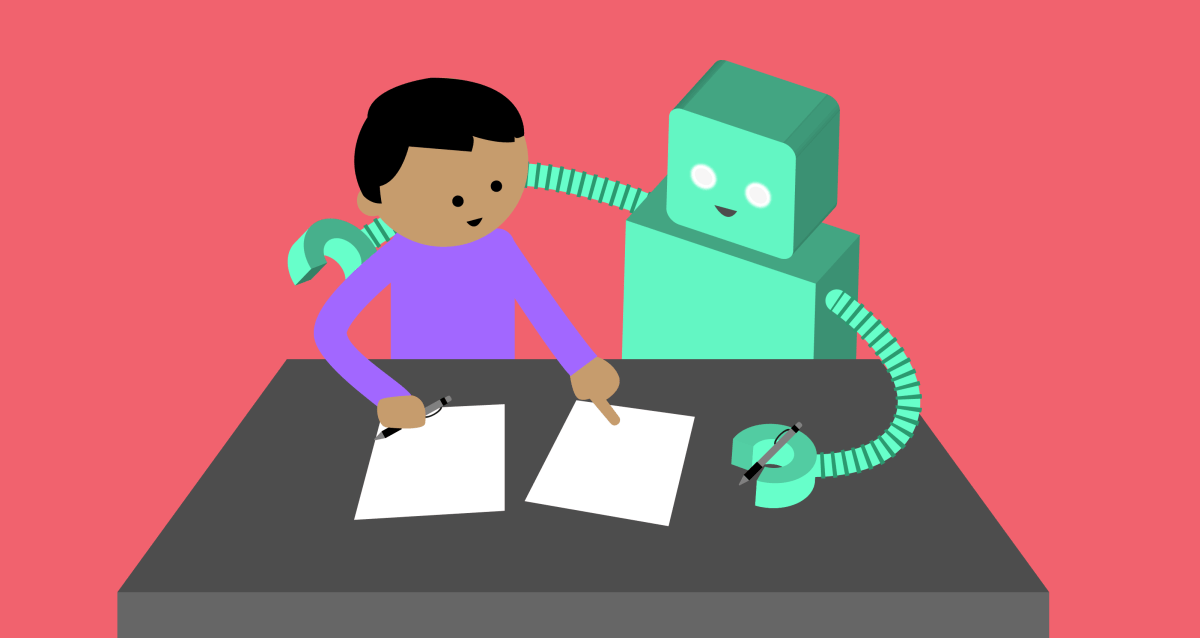