Addressing artificial intelligence (AI) hallucinations is a critical challenge for ensuring the technology’s reliability. A recent study suggests that multi-level agent systems, combined with natural language processing (NLP)-based frameworks, could significantly mitigate this issue.
In the study "Hallucination Mitigation using Agentic AI Natural Language-Based Frameworks," Gosmar and Dahl developed a three-tier agent system, testing its effectiveness in reducing hallucinations using 310 specially designed prompts. The results indicate that incorporating a second-tier agent reduced the hallucination score (THS) from -1.52 to -14.12, while implementing the third-tier agent further lowered it to -43.27 compared to the first level.
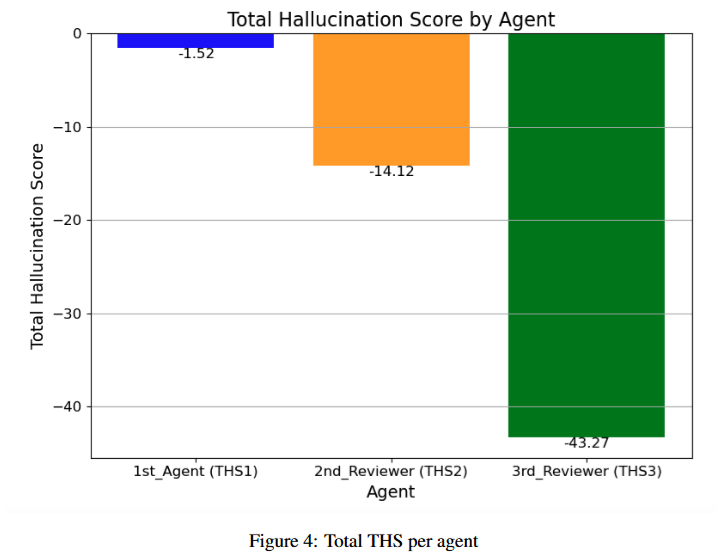
The authors evaluated the system’s effectiveness using four key performance indicators (KPIs): Factual Claim Density (FCD), measuring the concentration of factual statements; Factual Grounding References (FGR), tracking the number of fact-based citations; Fictional Disclaimer Frequency (FDF), assessing how often the system flags potentially fabricated content; and Explicit Contextualization Score (ECS), quantifying the extent of explicit contextualisation in responses.
The findings highlight a strong correlation between reducing hallucinations and the structured transfer of information between agents. The Open Voice Network (OVON) framework and structured data transmission formats, such as JSON messages, play a pivotal role in this process, enabling agents to identify and flag potential hallucinations effectively. This approach has not only proven successful in lowering hallucination scores but has also contributed to enhancing the transparency and reliability of responses.
Sources:
1.
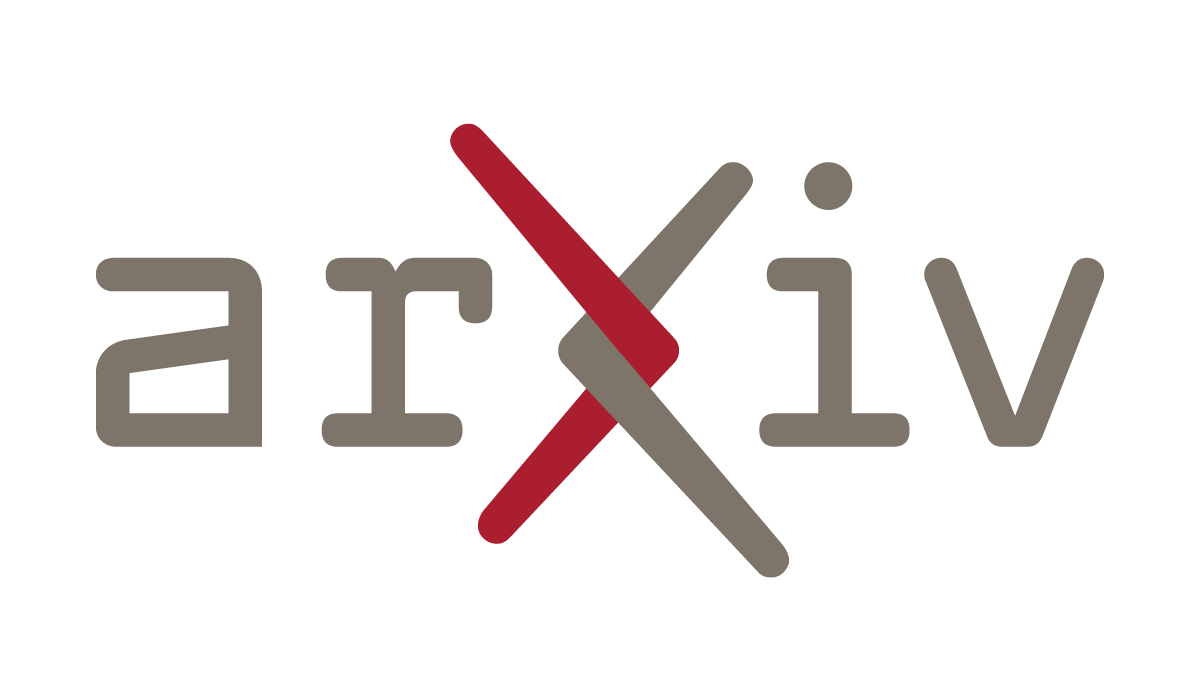