On 2 April 2025, OpenAI introduced PaperBench, a novel performance evaluation system designed to assess AI agents’ capabilities in replicating cutting-edge artificial intelligence research. Developed as part of the OpenAI Preparedness Framework, which measures AI systems’ readiness for complex tasks, PaperBench specifically challenges AI agents to accurately replicate 20 significant studies from the 2024 International Conference on Machine Learning (ICML). This involves understanding the research, coding, and conducting experiments.
PaperBench introduces a unique approach to measuring AI performance by breaking down the replication of each ICML 2024 study into 8,316 individually assessable micro-tasks, graded against detailed evaluation criteria developed in collaboration with the original authors. In evaluations, the top-performing agent, Claude 3.5 Sonnet equipped with open-source tools, achieved an average replication score of 21.0%. In comparison, when top-tier machine learning PhD students attempted a subset of PaperBench tasks, the results indicated that current AI models have not yet surpassed human performance in these tasks.
OpenAI has made the PaperBench code publicly available, encouraging further research into AI agents’ engineering capabilities. The SimpleJudge automated evaluation system, powered by large language models, achieved an F1 score of 0.83 on the JudgeEval test, significantly streamlining objective performance assessments of AI agents. The open-source initiative aims to enhance understanding of AI research replication and development, particularly as other models, such as GPT-4o and Gemini 2.0 Flash, scored notably lower at 4.1% and 3.2%, respectively.
Sources:
1.
2.
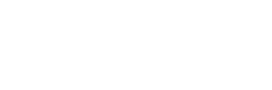
3.