Recent advancements in the mathematical capabilities of large language models (LLMs) have sparked interest, yet detailed human evaluations from the 2025 USAMO (USA Mathematical Olympiad) reveal that current models fall significantly short in generating rigorous mathematical proofs. While benchmarks like MathArena paint a positive picture of LLM performance on the AIME competition, where Gemini-2.5 Pro achieved results comparable to top human competitors, these assessments focused solely on the correctness of final numerical answers, overlooking the quality of rigorous reasoning and proof construction.
Expert evaluations of the six 2025 USAMO problems delivered sobering results: Gemini-2.5 Pro scored only 25%, while other models achieved less than 5%. According to the study Comparative Evaluation of LLMs’ Mathematical Reasoning, model performance on IMO-level problems was similarly poor, with correct solution rates ranging from 3.8% (DeepSeek) to 0% (Gemini 2.0). Evaluators identified common error types, including proof by example, unverified claims, and stating incorrect facts. As researchers noted, LLMs often rely on heuristics, shortcuts, and unfounded guesses, frequently leading to errors instead of rigorous reasoning.
The evaluations also highlighted that even when models produced correct final answers (occurring in 63.2% of cases for DeepSeek), the underlying reasoning was typically flawed. Models exhibited problematic patterns, such as citing non-existent sources and struggling to distinguish between correct and incorrect solutions. These findings clearly indicate that claims about LLMs’ olympiad-level mathematical abilities are exaggerated, underscoring the need for significant improvements in reasoning and proof-generation capabilities before these models can reliably tackle complex mathematical problems.
Sources:
1.
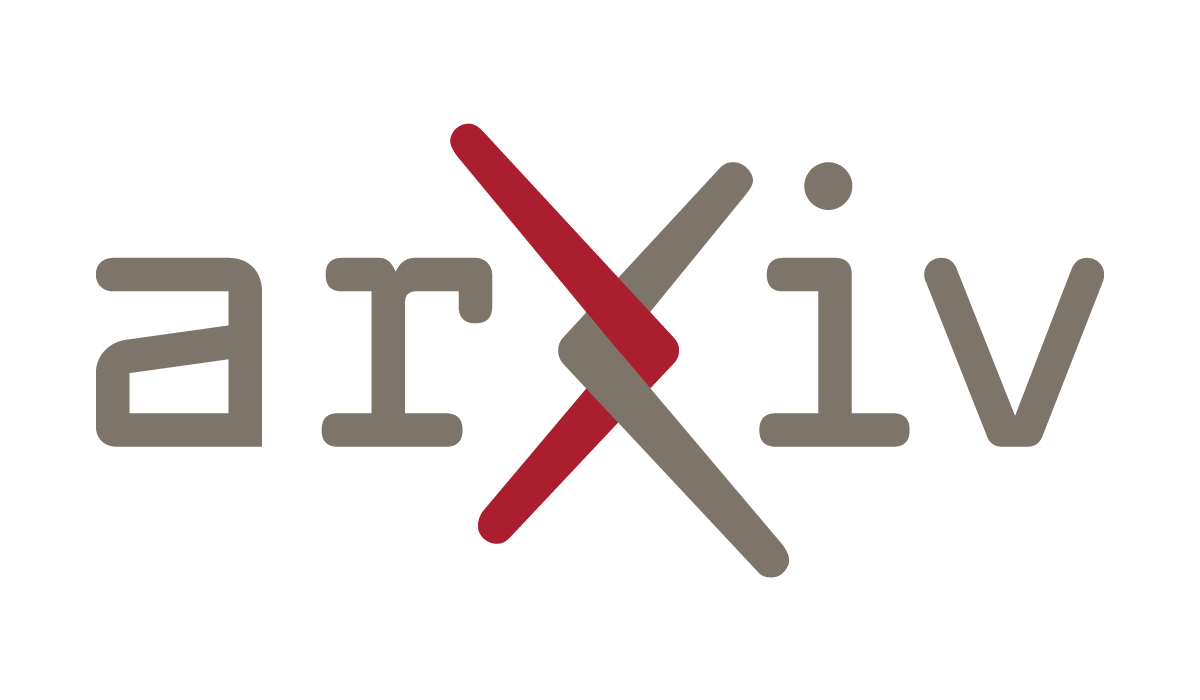
2.
3.
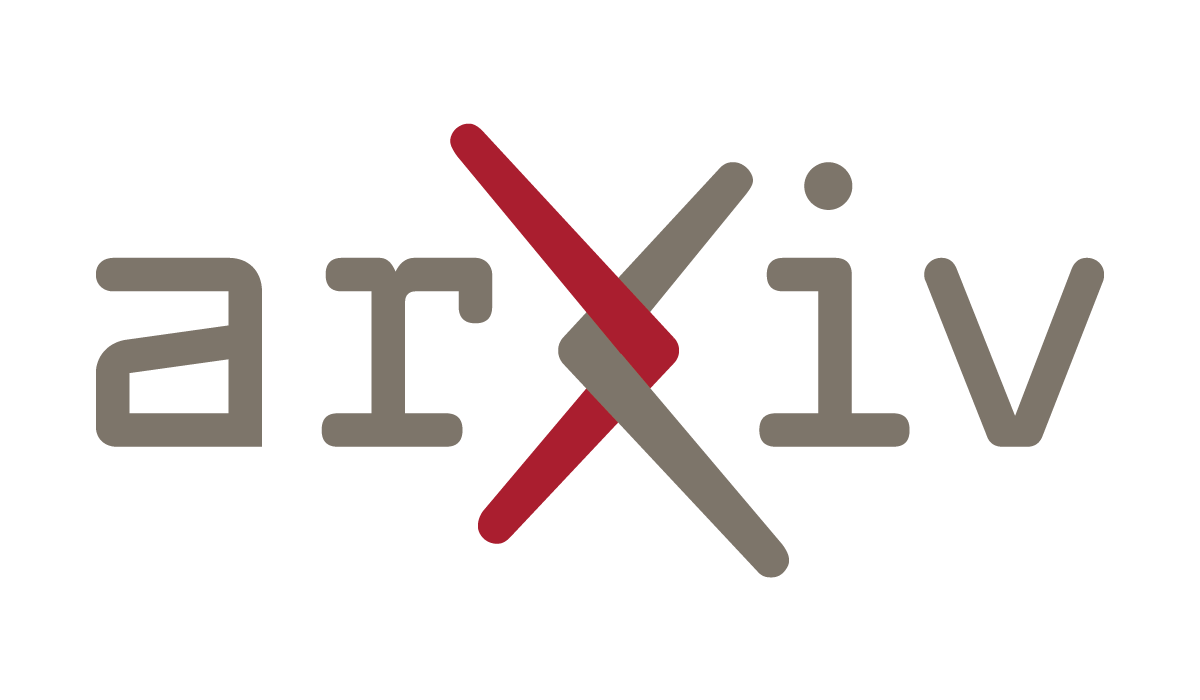